================ by Jawad Haider
07 - Saving and Loading Trained Models¶
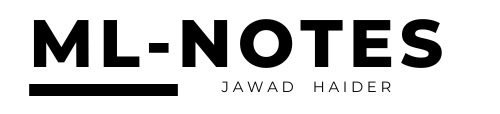
- Saving and Loading Trained Models
- Saving a trained model
- Loading a saved model (starting from scratch)
- That’s it!
Saving and Loading Trained Models¶
Refer back to this notebook as a refresher on saving and loading models.
Saving a trained model¶
Save a trained model to a file in case you want to come back later and feed new data through it.
To save a trained model called “model” to a file called “MyModel.pt”:
To ensure the model has been trained before saving (assumes the variables “losses” and “epochs” have been defined):
if len(losses) == epochs:
torch.save(model.state_dict(), 'MyModel.pt')
else:
print('Model has not been trained. Consider loading a trained model instead.')
Loading a saved model (starting from scratch)¶
We can load the trained weights and biases from a saved model. If we’ve just opened the notebook, we’ll have to run standard imports and function definitions.
1. Perform standard imports¶
These will depend on the scope of the model, chosen displays, metrics, etc.
# Perform standard imports
import torch
import torch.nn as nn
import numpy as np
import pandas as pd
2. Run the model definition¶
We’ll introduce the model shown below in the next section.
class MultilayerPerceptron(nn.Module):
def __init__(self, in_sz=784, out_sz=10, layers=[120,84]):
super().__init__()
self.fc1 = nn.Linear(in_sz,layers[0])
self.fc2 = nn.Linear(layers[0],layers[1])
self.fc3 = nn.Linear(layers[1],out_sz)
def forward(self,X):
X = F.relu(self.fc1(X))
X = F.relu(self.fc2(X))
X = self.fc3(X)
return F.log_softmax(X, dim=1)
3. Instantiate the model, load parameters¶
First we instantiate the model, then we load the pre-trained weights & biases, and finally we set the model to “eval” mode to prevent any further backprops.
model2 = MultilayerPerceptron()
model2.load_state_dict(torch.load('MyModel.pt'));
model2.eval() # be sure to run this step!
That’s it!¶
Toward the end of the CNN section we’ll show how to import a trained model and adapt it to a new set of image data.